Top Stories
Optimizing Fraud Management with AI Knowledge Graphs
Published : 6 years ago, on
The scope of fraud management for financial crimes has reached global proportions. Financial service organizations are contending with measures for Anti-Money Laundering (AML),Suspicious Activity Reports (SAR), counterfeiting and social engineering falsities, as well as synthetic, first-party, and card-not-present fraud. Such activities have spanned national and international borders.
The visual capabilities of graph analytics are ideal for monitoring, categorizing, and predicting threats to maximize prevention efforts. Graph techniques such as cluster analytics enable fraud management teams to visualize the distribution and acuteness of specific actors or fraud instances.
Supplementing this approach with Artificial Intelligence, however, provides the pivotal difference between merely monitoring fraud and impeding it. Machine learning algorithms can determine the probability of a particular fraud instance, codify it according to imminence or importance, then help issue alerts for preemptive action.
By compiling fraud-related data into an AI knowledge graph, risk management personnel can also triage those alerts for the right action at the right time. They also get the additive benefit of reusing this graph to decrease other risks for security, loans, or additional financial purposes.
Visualizing Fraudulent Behavior
Jan Aasman
Graph aware analytics represent relationships in ways users intuitively understand by illustrating connections between data and business objectives like fraud prevention. This visual approach is an integral aspect of investigating fraud, in which it’s crucial to understand relationships between different parties or events. Organizations should populate risk management knowledge graphs with as much data as possible about customers, including data about their customers. For example, when monitoring dollar clearing activities between an international bank in Paris and its Manhattan branch, these graphs should contain data about the parent company’s customers too.
This data form the basis for customer profiles predicated on user behavior, which are further codified into business attribute defined customer or event segmentation. These customer and event segments enable users to deploy visual techniques like cluster analytics to see a variety of relationships related to fraud, including clusters within segments for specific fraud types or macrocosms of inter-cluster relationships. The result is an effective fraud threat map; competitive visualization frameworks facilitate dynamic clustering without writing code.
The Machine Learning Effect
By incorporating AI, these threat maps yields a plethora of information for actually preventing fraud. Supervised learning methods can readily identify what events constitute fraud and which don’t; many of these involve classic machine learning.Unsupervised learning capabilities are influential in determining normal user behavior then pinpointing anomalies contributing to fraud. Perhaps the most effective way AI underpins risk management knowledge graphs is in predicting the likelihood—and when—a specific fraud instance will take place. Once organizations have data for customers, events, and fraud types over a length of time (which could be in as little as a month in the rapidly evolving financial crimes space), they can compute the co-occurrence between events and fraud types.
Co-occurrences are a core machine learning concept indicating how often events appear together. For example, fraud managers could compute the co-occurrence between the reissuing of a payment card and unauthorized ATM transactions. With the requisite data, they can even do so for particular customers. When co-occurrences are statistically significant according to the distribution of events, users can convert them to odds ratios to reveal their probability. Ascertaining the co-occurrence between various customers, events, and fraud instances can be as extensive as an organization likes, encompassing a range of variables. This process can also leverage reinforcement learning techniques and others in which the output of machine learning is input into the graph as knowledge for further analysis about when and how threats will occur.
Triaging Alerts
Action is derived from AI knowledge graph analytics in the form of alerts, which organizations can use to inform relevant parties about the likelihood of fraud occurring. Savvy organizations will triage those alerts so parties understand which fraudulent instance is likely to take place before others. Once alerts are issued, these platforms also offer germane information for other aspects of dealing with fraud such as impact analysis. They can identify exactly who the stakeholders are in the event of fraud, which in turn proves useful for effecting damage control. For the dollar clearing use case mentioned above, these risk management graphs can denote which customers of the French parent company are affected by any potential money laundering activity, for example.
Preemptive Action
Nonetheless, the true benefit of the AI knowledge graph approach to mitigating fraud lies in the preventative capabilities of these technologies. By calculating the probability of future fraudulent events manifesting, these platforms give organizations a critical temporal advantage for preventing them. Triaging alerts based on those probabilities is the first step to optimizing that time to forestall these financial crimes. With enough data, these graphs can provide similar benefits for securing loans or monitoring enterprise security.
About the Author:
Jans Aasman is Ph.D. psychologist and expert in Cognitive Science – as well as CEO of Franz.com, an early innovator in Artificial Intelligence and provider of Semantic Graph Databases and Analytics. As both a scientist and CEO, Dr. Aasman continues to break ground in the areas of Artificial Intelligence and Semantic Databases as he works hand-in-hand with organizations such as Montefiore Medical Center, Blue Cross/Blue Shield, Siemens, Merck, Pfizer, Wells Fargo, BAE Systems as well as US and Foreign governments.
Dr. Aasman spent a large part of his professional life in telecommunications research, specializing in applied Artificial Intelligence projects and intelligent user interfaces. He gathered patents in the areas of speech technology, multimodal user interaction, recommendation engines while developing precursor technology for the iPad and Siri from 1995 to 2004. He was also a part-time professor in the Industrial Design department of the Technical University of Delft.
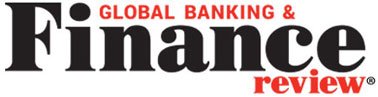
-
Finance3 days ago
Phantom Wallet Integrates Sui
-
Banking4 days ago
Global billionaire wealth leaps, fueled by US gains, UBS says
-
Finance3 days ago
UK firms flag over $1.4 billion in labour costs from increase in national insurance, wages
-
Banking4 days ago
Italy and African Development Bank sign $420 million co-financing deal