Banking
AI in Banking: How can legacy banks avoid falling behind?
Published : 2 weeks ago, on

Daniel Telling, Chief Growth Officer, Dufrain
By Daniel Telling, Chief Growth Officer at Dufrain
Artificial Intelligence (AI) is everywhere. It’s in the mainstream media, presidential election campaigns, and if you’re in Japan, it’s even in the public bathrooms. But as AI awareness and popularity grows, the pressure is mounting on organisations to not only keep pace, but to also leverage AI throughout all operations for an enhanced level of service. For banks, the stakes are particularly high. But what does AI mean for them, and how can they unlock its full potential?
First, it’s crucial to distinguish between the various types of AI. Technologies like Machine Learning (ML) and decision intelligence, which have been used in banking for decades to improve processes like fraud detection and risk analysis for example, are well established. The current buzz, however, revolves around Generative AI and Large Language Models (LLMs). While related, they are distinct technologies with their own capabilities and challenges.
When we talk about AI as a “game-changer,” we are generally referring to the combination of Generative AI and advanced ML. These innovations have the potential to reshape day-to-day operations, creating new efficiencies and better products with data at their core.
The Opportunities: Data and Automation
In an age where data is becoming a company’s most valuable asset, financial institutions that can effectively manage and leverage their customer information are well positioned to reap significant rewards. By building the right infrastructure, banks can unlock a range of services that benefit both internal and external customers.
Automated Decision Intelligence, for instance, can be leveraged to build smarter, more intuitive tools for customers and employees. These tools can help customers track spending, suggest budgeting strategies, and even predict future financial needs, creating a more proactive banking experience. AI tools such as GitHub’s Co-pilot can significantly reduce development cycles, enabling faster deployment of new services and products. This gives banks a competitive edge in an industry where speed and innovation are crucial.
However, while awareness is high, use cases in the traditional banking sector remain low. Capgemini Research Institute’s World Retail Banking Report 2024 found that only 6 per cent of retail banks are ready with a roadmap for enterprise-wide, AI-driven transformation at scale, and 3 per cent do not currently monitor AI key performance indicators at all.
The Challenges: Legacy Systems and Data Silos
Challenger banks currently have the advantage. They have been built with a ‘data-first’ mindset and integrate data throughout their entire operations. While for the traditional, incumbent banks, the transition from legacy systems to AI-powered solutions is a difficult journey. Many established banks face significant hurdles, including outdated infrastructure and siloed data that make it hard to leverage AI to its full potential.
The key to overcoming these challenges lies in three areas: foundations, service, and product.
Foundations: The first step in integrating AI into banking operations is ensuring that the right data infrastructure is in place. Legacy systems can often suffer from data quality issues due to poor governance, missing information, or siloed databases. Similarly, banks need to understand the data that they are exposing to AI, while also securing the data that they do not want to be part of the AI models. If unaddressed, these data challenges can severely limit the effectiveness of AI and automation. If data is fragmented or incomplete, for example, AI models cannot provide the insights needed to drive innovation or improve service delivery.
Services: Next, banks must think about how AI can transform the services they offer. AI’s impact isn’t limited to backend automation, it also changes how banks interact with both customers and employees. For example, business intelligence teams need to be equipped with the right tools to analyse AI-driven insights, while customer-facing teams must be trained to leverage these insights in real time.
Product: Finally, AI needs to be integrated into the entire product development process, and products should be developed with a ‘data-first’ mindset. For banks to offer truly innovative financial products, the right data must be embedded in all product design. Collaboration between data and product teams is essential to ensure that AI is not just an add-on but a foundational element. This integration will enable the creation of products that are not only more responsive to customer needs but also more adaptive in a rapidly changing market.
Harnessing data for maximum reward
For banks, the adoption of AI is not a question of if, but how and when. While the opportunities are vast, the challenges should not be underestimated. Legacy systems, data silos, and organisational resistance can stand in the way of a bank reaching AI’s full potential.
The key for banks will be to lay the right foundations. By starting with robust data practices, having a clear strategy for service innovation, and integrating AI into their product development processes from the beginning, they can harness the game-changing potential of AI and stay ahead in an increasingly competitive market.
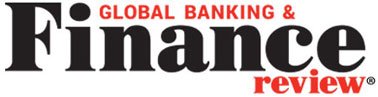
-
Finance3 days ago
Phantom Wallet Integrates Sui
-
Banking4 days ago
Global billionaire wealth leaps, fueled by US gains, UBS says
-
Finance3 days ago
UK firms flag over $1.4 billion in labour costs from increase in national insurance, wages
-
Banking4 days ago
Italy and African Development Bank sign $420 million co-financing deal