Technology
Navigating AI Bias: Ensuring Data Integrity in the Age of Generative AI
Published : 2 months ago, on
Tendü Yogurtcu
By Tendü Yogurtcu, PhD, chief technology officer at Precisely
Since the emergence of generative artificial intelligence (AI), the business landscape has transformed into a new world of efficiency and innovation. Many view generative AI as the ultimate silver bullet in organisations’ arsenals – it streamlines processes, discovers new avenues for innovation, analyses masses of data within seconds, and even personalises the customer experience.
This wide array of benefits has led to 34 percent of UK businesses implementing at least one form of artificial intelligence into their systems in 2024, and 59 percent have indicated that their spending on AI projects will rise compared to 2023.
However, despite its popularity, the use of AI does not always guarantee success. Many organisations have implemented it into their systems without adequately preparing their data and processes, leading to a minefield of issues.
When AI goes wrong
Although it is perceived as impartial, the reality is that AI is a product of the data that fuels it. For example, if there is a lack of representation in the data powering an AI model, it is highly likely to start producing biased outputs. In fact, AI bias – the production of incorrect outputs due to inaccurate, incomplete, or unreliable data – is one of the most prominent AI-related issues facing modern businesses. For many organisations, their data is either living in silos, is stale, unstandardised, is full of duplicates, or lacks the insight required to make it usable. This translates into a range of issues, such as irrelevant or inaccurate outcomes, which can lead to significant real-world consequences.
For example, in the financial services industry, AI is often used for credit scoring to determine the creditworthiness of loan applicants. However, if the data used to train the AI model is biased, it can lead to unfair outcomes. For instance, if the historical data includes a disproportionate number of loan defaults from a particular demographic group, the AI model might learn to associate that group with higher risk. As a result, the model could unfairly deny loans to individuals from that demographic group, even if they are financially stable and creditworthy.
This is already occurring in the real world, in fact one financial institution’s AI system was found to be biased against women. The system was trained on historical data that reflected existing gender biases in lending practices. Consequently, women were more likely to be denied loans or offered less favourable terms compared to men with similar financial profiles. This bias not only perpetuated existing inequalities but also harmed the financial institution’s reputation and led to regulatory scrutiny.
Ultimately, as AI is integrated into all aspects of the business world, organisations have a responsibility to ensure it is accurate and reliable, as the ramifications can be detrimental. Underpinning these tools with trustworthy data is critical for any organisation looking to capitalise on the endless capabilities that AI can offer. Consequently, many organisations are looking to boost their data integrity by prioritising data integration, data quality and governance, location intelligence, and data enrichment.
Break down silos with data integration
Many organisations are vulnerable to AI bias as they have an array of data living in different systems, stored in different formats. When data is siloed across lines of businesses and across data platforms, it is extremely difficult to create an accurate and unified view of the organisation’s data. As a result, the AI’s outputs may not fully reflect the available information, potentially leading to ineffective recommendations, such as marketing campaigns that fail to consider the latest point of sales data.
By integrating critical data across cloud, on-premises, and hybrid environments as well as across business functions, organisations can help ensure that data is integrated, complete, consistent, and accurate – improving the reliability of AI results and reducing the risk of errors and biases.
Build robust frameworks for data quality and governance
Collating wide ranges of data and translating it into one format is not enough by itself to prevent AI bias. Data can still be inaccurate, inconsistent, missing, or containing duplicates. Consequently, including all relevant and critical data is only the first step – organisations must also look to ensure the quality and governance of the data fuelling AI models.
A robust data quality strategy should include tools that can monitor health of data on an ongoing basis, cleanse, de-duplicate, and validate critical data, whilst also producing dashboards and automated workflows. This helps companies to proactively observe, detect, and address data quality issues faster and with less difficulty.
Data governance helps align technology, people, and processes, enabling organisations to have a wider understanding of its data. This creates enhanced visibility, which strengthens the accountability and quality of an organisation’s data assets and allows it to be correctly monitored to ensure compliance with privacy and security regulations. By combining data quality and governance frameworks, organisations can look to considerably enhance the extent of which trustworthy insights can be drawn from their data and AI models. Data governance is also a key part of AI governance.
Enrich data for contextual relevance
Regardless of whether data is accurate or complete, if it lacks context its outputs will be vulnerable to biases, as it won’t be able to consider the subtle and complex details that could alter the value of the data. For example, if AI was used to predict flight demand across a calendar year and the data it used included the year of the COVID pandemic – without the correct context around it, the data would not be an accurate representation of normal flying habits. Therefore, for data to lack bias, it must also include context to eliminate the impacts of anomalies.
Enriching data with trustworthy third-party datasets and geospatial insights can help companies to improve the diversity of their data whilst also revealing any undiscovered patterns that could have been previously overlooked. Datasets that enhance these insights include demographics data, detailed address information, consumer behaviour, points of interest data, and environmental risk factors.
When AI is fuelled by contextual data, organisations can be confident that they are facilitating the most relevant and reliable outputs for all possible applications, whilst minimising the likelihood of data bias.
Data integrity is the key to AI success
Organisations are seeing AI as the new gold rush of the technology world, making it the ultimate way to remain competitive. However, as AI deployment grows, so will the number of organisations that suffer from AI-related issues, including bias. Mitigating bias requires taking action for de-biasing the data as well as mitigating algorithmic bias and systematically inspecting algorithmic decision making.
As a result, organisations must ensure that the data used to train their AI models and the data used for AI predictions is reliable. If the data is inaccurate or irrelevant, it can lead to significant repercussions, including regulatory fines and reputational damage.
Now more than ever, organisations need to be proactive when it comes to building a meaningful, sustainable data strategy that combines data integration, data governance and quality, location intelligence, and data enrichment. By doing so, they can use AI and generative AI to accelerate data-driven decisions.
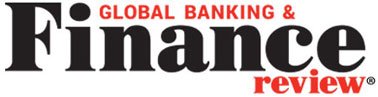
-
Finance3 days ago
Phantom Wallet Integrates Sui
-
Banking4 days ago
Global billionaire wealth leaps, fueled by US gains, UBS says
-
Finance3 days ago
UK firms flag over $1.4 billion in labour costs from increase in national insurance, wages
-
Banking4 days ago
Italy and African Development Bank sign $420 million co-financing deal